Module Overview
Statistical tests are used to determine whether the observed effect is real or merely occurred by chance. An outcome of a statistical test is a p-value, the probability of getting the observed, or stronger, result by chance. P-values are essential for data analysis, but alas, they are frequently misunderstood and misused, often considered as the ultimate answer, while they only form a small part of the bigger puzzle. This ultimately results in the publication of erroneous and irreproducible results.
This series of lectures explores a range of statistical tests, when their use is appropriate and correctly interpreting their results. The topics covered by the lectures are as follows:
- Introduction: The null hypothesis, statistical tests, p-values; Fisher’s test
- Contingency tables: chi-square test, G-test
- T-test: one- and two-sample, paired; variance comparison
- ANOVA: one- and two-way
- Non-parametric tests: Mann-Whitney, Wilcoxon signed-rank, Kruskal-Wallis
- Non-parametric tests: Kolmogorov-Smirnov, permutation, bootstrap
- Statistical power: Effect size, power in t-test, power in ANOVA
- Multiple test corrections: Family-wise error rate, false discovery rate, Holm-Bonferroni limit, Benjamini-Hochberg limit, Storey method
- What’s wrong with p-values?
Pre-registration is not required to attend this lecture series.
Learning Outcomes
- Be able to select an appropriate statistical test to apply
- Understand how to interpret p-values and false discovery rates
- Understand when use of a p-value is appropriate
Prerequisite Modules/Knowledge
Course Schedule
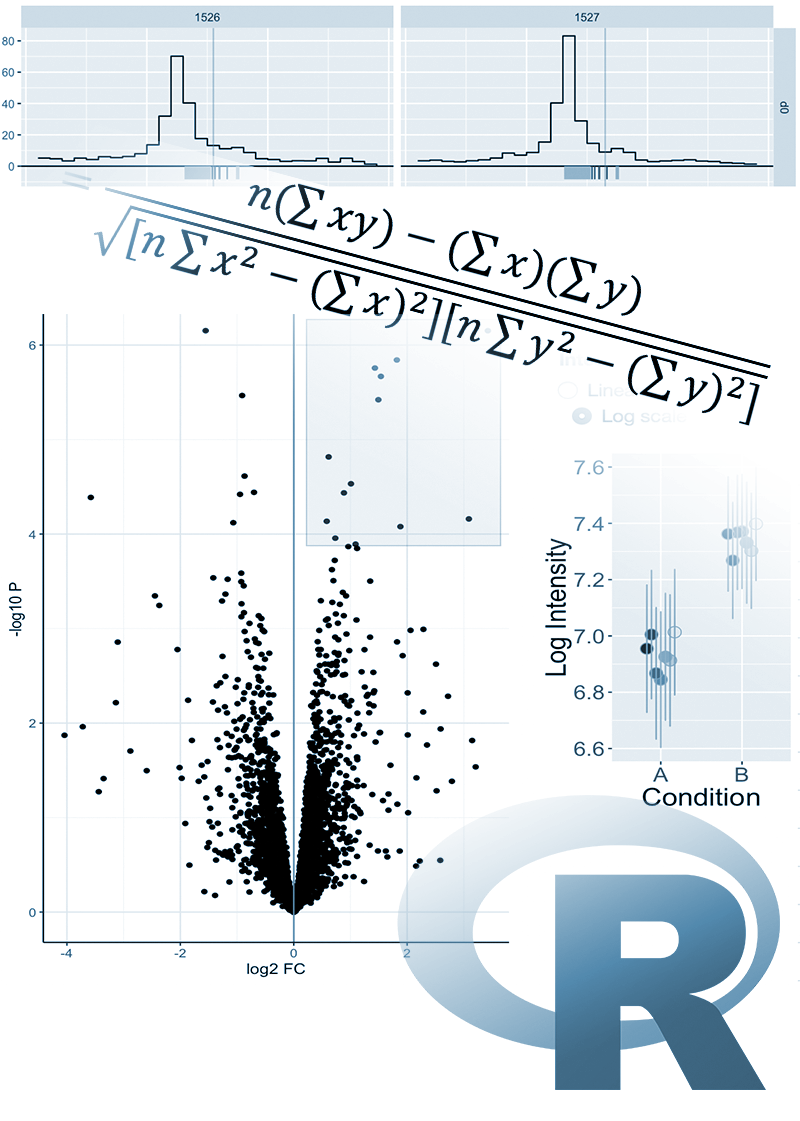